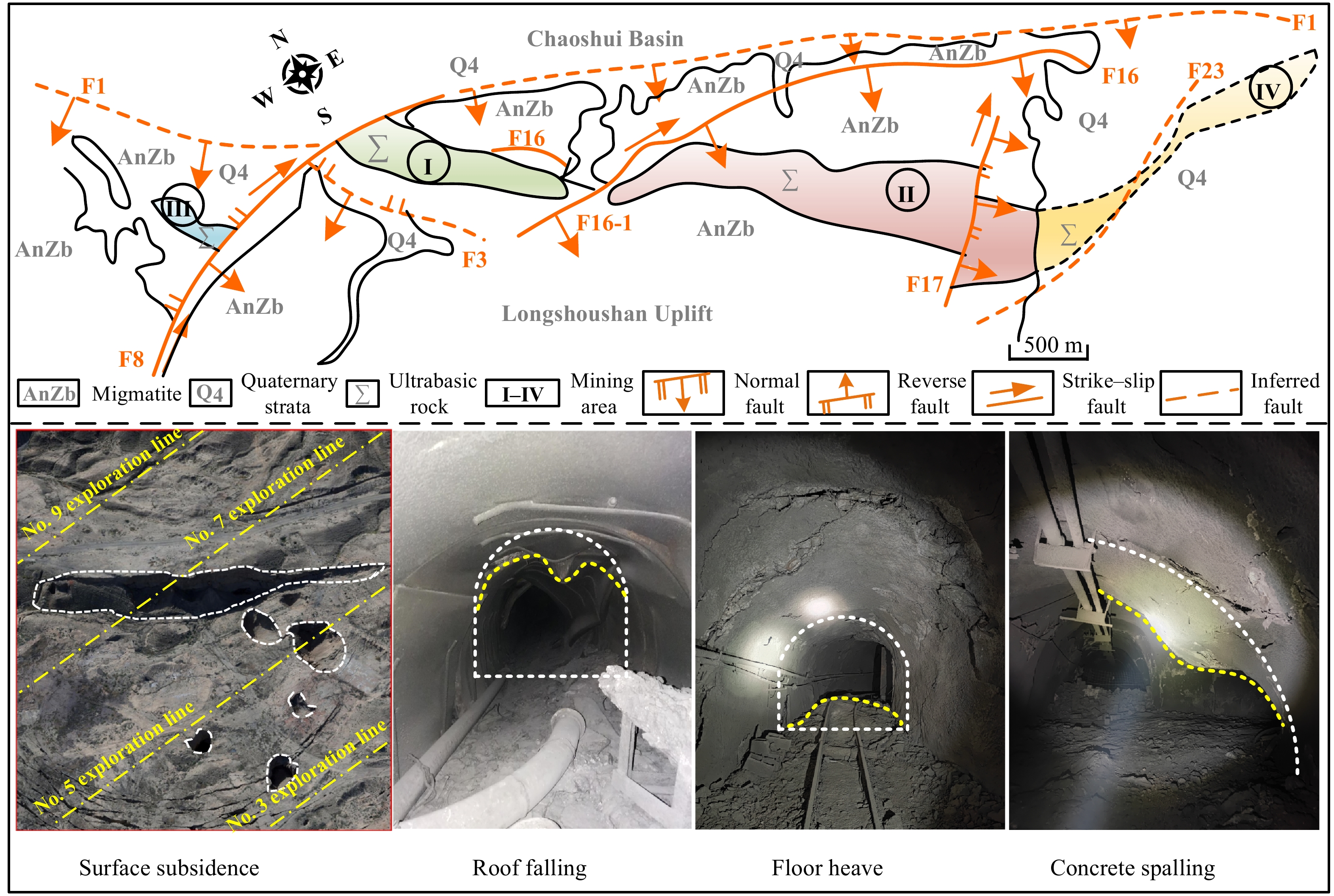
Cite this article as: | Dapeng Chen, Shenghua Yin, Weiguo Long, Rongfu Yan, Yufei Zhang, Zepeng Yan, Leiming Wang, and Wei Chen, Heterogeneous information phase space reconstruction and stability prediction of filling body–surrounding rock combination, Int. J. Miner. Metall. Mater., 31(2024), No. 7, pp.1500-1511. https://dx.doi.org/10.1007/s12613-024-2916-6 |
The filling mining method is a recent trend in underground mining and is also a development requirement for green mines [1–3]. In the process of filling mining from shallow to deep in broken metal mines, a large area of filling body is formed. During the mining process and after filling the mined-out area, it is important to monitor the load-bearing characteristics of the filling body, which are key to determining the stability of the stope [4–5]. Due to multiple fillings as well as differences in filling quality and interval filling, there are intricate weak surfaces within the large filling body, along with residual mined-out areas where the filling does not connect to the roof. Under complex stress conditions, a poor geological body consisting of filling bodies may cause large-scale underground destabilization accidents. Therefore, it is important to evaluate the stability of the filling body–surrounding rock combination to ensure operational safety during underground resource extraction.
The stability monitoring of filling bodies and rock masses mainly includes two areas: surface and underground. Surface monitoring methods include global positioning system and real-time kinematics (GPS-RTK) [6], interferometric synthetic aperture radar (InSAR) [7–9], and laser scanning [10–11]. Underground monitoring equipment includes optical fiber monitoring [12–14], multipoint displacement meter [15], inclinometer [16–17], and microseismic monitoring system [18–19]. There are many methods to evaluate the stability of filling body and rock mass, including the deep learning method to process microseismic signals to realize the risk prediction of mine rock burst [20–21] and the acoustic emission early warning model based on catastrophic theory [22]. However, the process of assessing the stability of the filling body and the surrounding rock is affected and controlled by several uncertain factors. Furthermore, the analysis of a certain factor alone is often biased and half-assed. Therefore, quantifying the stability of the filling body by comprehensively considering the coupled influence of multiple factors and parameters is of great significance.
At present, the evaluation system of filling body–surrounding rock combination has not been established. The large-area filling body is affected by multiple filling, interval filling, and filling quality. Apart from the complex contact mode between filling bodies and between filling bodies and surrounding rock, the rock’s mechanical properties also exhibit a high degree of nonlinearity [23–26]. Current research has faced difficulties in accurately evaluating and predicting the stability of filling body–surrounding rock combinations.
Jinchuan Group’s Longshou Mine (located in Gansu Province, China) adopts a downward layered cemented filling mining method, in which mining operations are carried out under artificial false roofs after filling. Compared with the case of a fully connected roof, the energy storage capacity of the filling body–surrounding rock combination without a fully connected roof is weak, and the ability to resist the internal damage and energy release caused by stress concentration is also poor [27–28]. Thus, due to the uncertainty in the filling quality, there is a possibility of the roof of the filling body collapsing during the mining process [29–30]. When the filling body collapses, there is a greater safety risk during treatment, as well as a potential safety hazard for the next sublevel of the mining operation [31–35].
As depicted in Fig. 1, Longshou Mine is a typical high-stress mine, where faults and joints are developed, and the horizontal tectonic stress is as high as 50 MPa. In 2016, a collapse of the overlying filling body–surrounding rock combination in the West II mining area of the Longshou Mine occurred—a relatively rare event in the history of filling mining [36]. This accident has subverted the traditional concept that the filling bodies can effectively manage the ground pressure. In fact, the stope using the filling mining method also has the risk of instability and collapse, which greatly threatens production safety in the mine location.
To solve the above problems, this paper proposes a phase space reconstruction and stability prediction method for processing heterogeneous information of filling body–surrounding rock combinations. A time series (i.e., heterogeneous information time series) containing multiple monitoring data is obtained by constructing a three-dimensional (3D) monitoring system of a large backfill–surrounding rock combination. Upon combining the average mutual information method and the false nearest neighbor point method, the heterogeneous information time series of the filling body–surrounding rock combination is reconstructed and mapped into the phase space. The distance between the phase point and its nearest point is used as the evaluation distance to characterize the change in the stability state of the measuring point. This method is then used to analyze the evolution trend of the stability state of measuring points of each level in the middle mining area based on the background of Longshou Mine. At the same time, the prediction performance of the autoregressive integrated moving average (ARIMA) model and machine learning models, along with long short-term memory (LSTM) and Transformer, on the evaluation distance is discussed, and the feasibility of this method is verified.
The ore body of Longshou Mine is deeply buried, and the in-situ stress is high. Therefore, the mine adopts the hexagonal layered downward cemented filling mining method, which is carried out by implementing interval mining and pipeline artesian filling. As the depth of mining increases, the risk of underground mining operations increases sharply. Unfavorable factors such as high underground pressure and rock mass fragmentation may induce disasters, including large-scale rock mass movement and overall instability of the filling bodies.
To monitor the stability of filling bodies and surrounding rock mass, a deep 3D monitoring system in Longshou Mine has been built. In this system, we used inclinometers, borehole stress meters, and multipoint displacement meters to carry out the dynamic monitoring of typical layered overlying filling bodies and surrounding rock mass in the middle mining area and West I mining area. This work can provide data support for stability evaluation and disaster warning of overlying filling bodies in deep filling stopes.
As shown in Fig. 2, combined with field investigation and numerical simulation analysis, the potential instability areas of overlying filling bodies in underground stopes are determined. We used borehole imaging to collect the surrounding rock joint data of the orebodies and the backfill, after which we analyzed the stability of the rock mass at each level by using the engineering rock mass basic quality classification (BQ) method. Based on the mining history of Longshou Mine, the authors simulated and analyzed the distribution of the plastic zone, stress field, and displacement field of the surrounding rock mass after mining.
The numerical simulation results reveal that the potential instability areas of the filling body–surrounding rock combination are mainly distributed in the combination of the backfill bodies and surrounding rock. The plastic zone is distributed in the surrounding rock of the filling bodies, and fill mining results in large-scale stress concentrations at the contact area between the filling bodies and the surrounding rock. Furthermore, the displacement in the contact surface area is significantly greater than in the inner part of the filling bodies. Therefore, in this paper, the areas with poor surrounding rock quality and located in the combination of filling bodies and surrounding rock are regarded as the key monitoring locations.
We selected six different exploration lines at different levels in the middle mining area and West I mining area of Longshou Mine to carry out the monitoring tests. The distribution of measuring points at each level is shown in Table 1 and Fig. 3. The monitoring points involved in this 3D monitoring system are located in areas where the quality of the rock mass is classified as IV and V.
Measuring points ID | Altitude / m | Location |
1 | 1500 | No. 22 exploration line |
2 | 1485 | No. 25 exploration line |
3 | 1470 | No. 28 exploration line |
4–6 | 1460 | No. 21, No. 25, and No. 33 exploration lines |
7–9 | 1280 | No. 14, No. 21, and No. 27 exploration lines |
10–12 | 1220 | No. 21, No. 25, and No. 29 exploration lines |
Three types of monitoring instruments were used in this system, namely multipoint displacement meter (MDM), borehole stress gauge (BSG), and inclinometer, which are used to monitor the separation, stress change, and settlement information of filling body–surrounding rock combination, respectively. These instruments were installed in the filling body and surrounding rock through drilling. The installation information of each measuring point is shown in Table 2, while Fig. 4 shows the spatial position of the instruments. The multipoint displacement meters, which were arranged inside the filling bodies and the surrounding rock, were installed vertically downward by drilling boreholes at the bottom of the roadway. Three borehole stress gauges were arranged at each measurement point, corresponding to the strike, inclination, and vertical direction of the ore body, respectively. The inclinometer tubes were buried in horizontal boreholes. To accurately measure the displacement changes of the filling bodies–surrounding rock combination, the inclinometer tube must be in contact with both the surrounding rock and the filling bodies at the same time. Furthermore, the inclinometers were arranged uniformly inside the inclined tube to monitor the settlement at different locations relative to the mouth of the tube.
Instrument type | Number of installations | Description |
MDM | 1 | Two anchoring points |
BSG | 3 | Install in three mutually perpendicular directions |
Inclinometer | 1 | Inclinometer tube length 30–50 m |
The mechanical properties of rock mass and filling bodies are nonlinear because of the anisotropy of the component materials. The filling body–surrounding rock combination can be regarded as a nonlinear system under complex geological processes and mining disturbances. In the mines using the filling mining method, it is of great significance to parsing the stress, displacement, and other parameters of the filling body and surrounding rock to ensure proper underground safety management. In general, the deformation state data collected by the instruments is a nonlinear time series in the filling body–surrounding rock combination. In the current paper, these data were defined as multiple time series.
With the goals of realizing the fusion analysis of multiple sensor data, avoiding the influence of subjective factors in the process of monitoring data analysis, and improving the accuracy of the stability judgment of the filling body–surrounding rock combination, the authors used the average mutual information method [37] and the false nearest neighbor method [38] to construct the multivariate time series phase space of the filling body–surrounding rock combination. Phase space is an effective tool to describe the system movement and evolution, in which any system state can be represented by a point in the phase space. In the current paper, these monitoring data are mapped into the multidimensional phase space to approximate the recovery of the nonlinear relationship between the deformation states of the filling body–surrounding rock combination.
The average mutual information can be used to measure the interdependence of two time series. To explore as much information as possible about the dynamics of the time series, phase space reconstruction should be performed using the time lag corresponding to the minimum average mutual information.
The information entropy of the time series S = {x(t1),x(t2),⋯,x(tn)} and Q={x(t1+τ),x(t2+τ),⋯,x(tn+τ)} is calculated by:
H(S)=−∑ni=1PS(x(ti))log2PS(x(ti)) | (1) |
H(Q)=−∑nj=1PQ(x(tj+τ))log2PQ(x(tj+τ)) | (2) |
where PS(x(ti)) and PQ(x(tj+τ)) are the probabilities of events x(ti) and x(tj+τ) in sets S and Q, respectively.
When the time series S is known, the mutual information of S and Q is defined as
I(Q,S)=H(Q)−H(Q|S) | (3) |
where H(Q|S)=−∑i,jPSQ(x(ti), x(tj+τ))log2PSQ(x(ti),x(tj+τ)), in which PSQ(x(ti), x(tj+τ)) is the joint distribution probability of x(ti) and x(tj+τ).
Therefore, the mutual information of S and Q can be calculated as follows:
I(Q,S)=∑i,jPSQ(x(ti),x(tj+τ))×log2(PSQ(x(ti),x(tj+τ))PS(x(ti))PQ(x(tj+τ))) | (4) |
Obviously, I(Q, S) is a function related to the time lag τ, which is denoted by I(τ). Here, I(τ)=0 means that the time series S and Q are completely uncorrelated, and Q is completely unpredictable. In this case, having a minimum value of I(τ) may mean a maximum possible uncorrelation between the time series S and Q.
To improve the efficiency of time lag selection, referring to related studies [39–40], the authors of the current study used the τ corresponding to the first local minima of I(τ) as the time lag for phase space reconstruction, or the τ corresponding to when I(τ) is less than 1e.
In the process of mapping a heterogeneous information time series into a higher dimensional phase space, points that are adjacent in the lower dimensional space but discrete in the higher dimensional space are called “false neighbors.” As the embedding dimension increases, the false neighbor points in the phase space gradually decrease. When there are no false neighbor points in the phase space, the geometric structure of the time series can be considered completely opened, and the trajectory of the chaotic movement can be restored [38].
In a d-dimensional phase space, the vector of the phase point ti is {\boldsymbol{x}}_{{\boldsymbol{d}}}(t_i)=\left\{x(t_i),\ x(t_i+\tau),\ \cdots,\ x(t_i+(d-1)\tau)\right\} , its nearest neighbor is {\boldsymbol{x}}_{\boldsymbol{d}}^{\mathbf{N}\mathbf{N}}({t}_{i})=\{{x}^{\mathrm{N}\mathrm{N}}({t}_{i}),\;{x}^{\mathrm{N}\mathrm{N}}({t}_{i}+\tau ),\;\cdots ,\; {{\boldsymbol{x}}}^{\bf{N}\bf{N}} ({t}_{i}+(d-1)\tau )\} , and the square of the Euclidean distance [38] between them is given by:
{R}_{d}^{2}({t}_{i})= \sum\nolimits _{k=0}^{d-1}{\left[x({t}_{i}+k\tau )-{x}^{\mathrm{N}\mathrm{N}}({t}_{i}+k\tau )\right]}^{2} | (5) |
When the dimension of the phase space increases from d to d+1 dimensions, the distance [38] between {\boldsymbol{x}}_{\boldsymbol{d}{\boldsymbol{+1}}}({t}_{i}) and {\boldsymbol{x}}_{\boldsymbol{d}{\boldsymbol{+1}}}^{\mathbf{N}\mathbf{N}}({t}_{i}) can be calculated as:
{R}_{d+1}^{2}({t}_{i})={R}_{d}^{2}({t}_{i})+{\left[x({t}_{i}+d\tau )-{x}^{\mathrm{N}\mathrm{N}}({t}_{i}+d\tau )\right]}^{2} | (6) |
For a heterogeneous information time series containing D attributes, Eqs. (5) and (6) should be respectively rewritten as [40]:
{R}_{d\cdot D}^{2}({t}_{i})= \sum\nolimits _{j=1}^{D} \sum\nolimits _{k=0}^{d-1}{\left[{x}_{j}({t}_{i}+k\tau )-{x}_{j}^{\mathrm{N}\mathrm{N}}({t}_{i}+k\tau )\right]}^{2} | (7) |
{R}_{(d+1)\cdot D}^{2}({t}_{i})={R}_{d\cdot D}^{2}({t}_{i})+ \sum\nolimits _{j=1}^{D}{\left[{x}_{j}({t}_{i}+d\tau )-{x}_{j}^{\mathrm{N}\mathrm{N}}({t}_{i}+d\tau )\right]}^{2} | (8) |
If the difference between {R}_{(d+1)\cdot D} and {R}_{d\cdot D} is large, the two phase points can be considered as not adjacent in the higher dimensional phase space, which means that they are regarded as each other’s false nearest neighbors. The false nearest neighbor points can be judged by the following criterion [38,40]:
C(t_i,\ d) > R_{\tau} | (9) |
where C(t_i,\ d)=\dfrac{\sum_{j=1}^D\left[x_j(t_i+d\tau)-x_j^{\mathrm{N}\mathrm{N}}(t_i+d\tau)\right]^2}{R_{d\cdot D}} , which measures the difference in distance between two phase points in two phase spaces. In addition, the threshold {R}_{\tau } is generally chosen between \left[10,\mathrm{ }50\right] , and in this paper, its value is taken as 10. During the implementation of the false nearest neighbor method, the embedding dimension d is gradually increased, and the corresponding false nearest neighbor ratio is calculated at the same time. In this case, the geometric structure of the chaotic time series can be considered to be fully opened when the false nearest neighbor proportion is less than 5% or no longer decreases with the increase of embedding dimension.
The data from different sensors constitute a heterogeneous information time series. Here, we denote the time series with the number of time points N and the number of sensors M as {\boldsymbol X}=\left\{{\boldsymbol X}_{\boldsymbol{t}1},{\boldsymbol X}_{\boldsymbol{t}2},\ \cdots {\boldsymbol X}_{\boldsymbol{t}\boldsymbol{i}},\ \cdots,\ {\boldsymbol X}_{\boldsymbol{t}\boldsymbol{N}}\right\} , where i\in\{1, \ 2,\ \cdots,\ N\} . Each element in the time series \mathit{X} represents the dataset collected at each time point, denoted as {\boldsymbol X}_{\boldsymbol{t}\boldsymbol{i}}= \{{x}_{ti,1}, \; {x}_{ti,2},\;\cdots ,\;{x}_{ti,j},\;\cdots ,\;{x}_{ti,M}\} , where j\in\left\{\mathrm{1,\ 2},\ \cdots,\ M\right\} , and {x}_{ti,j} represent the value of the j th sensor at the moment {t}_{i} .
Based on the heterogeneous information time series, the data from each sensor are then used to reconstruct the integrated time series {\boldsymbol X}'=\left\{{\boldsymbol X}_{\boldsymbol{t}1}',\;{\boldsymbol X}_{\boldsymbol{t}2}',\ \cdots,\ {\boldsymbol X}_{\boldsymbol{t}\boldsymbol{i}}',\ \cdots,\boldsymbol{\ X}_{\boldsymbol{t}\boldsymbol{N}}'\right\} of heterogenous information in phase space with the appropriate time lag \tau and embedding dimension d. In addition, {\boldsymbol{X}}_{\boldsymbol{t}\boldsymbol{i}}' is the reconstructed time series of {\boldsymbol{X}}_{\boldsymbol{t}\boldsymbol{i}} and is expressed as:
{\boldsymbol X}_{\boldsymbol{t}\boldsymbol{i}}'=\left[\begin{array}{c}{x}_{ti,1},\;{x}_{ti-{\tau }_{1},1},\;\cdots ,\;{x}_{ti-({d}_{1}-1){\tau }_{1},1},\;{x}_{ti,2},\;{x}_{ti-{\tau }_{2},2},\;\cdots ,\;{x}_{ti-({d}_{2}-1){\tau }_{2},2},\;\cdots ,\\ {x}_{ti,j},\;{x}_{ti-{\tau }_{j},j},\;\cdots ,\;{x}_{ti-({d}_{j}-1){\tau }_{j},j},\;\cdots ,\;{x}_{ti,M},\;{x}_{ti-{\tau }_{M},M},\;\cdots ,\;{x}_{ti-({d}_{M}-1){\tau }_{M},M}\end{array}\right] | (10) |
where {\tau }_{j} and {d}_{j} are the time lag and embedding dimension of the j th attribute, respectively.
The trajectory of the integrated time series from the monitoring system in phase space shows the stability evolution of the filling body–surrounding rock combination. The distance between the phase points of the two adjacent time points represents the changing amplitude of the stability state of the measuring point. The ED is the distance between the corresponding phase point of the current time point in phase space and its closest point. It is an indicator that measures the maximum similarity between the stability states at the current moment and any other previous moment. The ED is proportional to the difference in the stability state between the two time points. When the evaluation distance increases, it indicates that the difference between the state of the current moment and the previous moment becomes larger and that there is a jump change in the stability of the system. That implies a risk of mutation in the stability of the filling body–surrounding rock combination. Therefore, in the present paper, the authors propose to use the ED as an indicator to characterize the degree of stability evolution of the filling body–surrounding rock combination. Meanwhile, the ARIMA model was applied to predict the ED time series.
In the integrated time series of heterogenous information, we calculate the ED of each phase point sequentially. If the nearest neighboring phase point of the phase point {\boldsymbol{X}}_{\boldsymbol{t}\boldsymbol{k}}' is {\boldsymbol{X}}_{\boldsymbol{t}\boldsymbol{b}}' , where k,\ b\in\left\{\mathrm{1,\ 2},\ \cdots,\ n\right\} and k\ge b , the Euclidean distance between them can be calculated using the following equation:
{Z}_{k}=\left|{\boldsymbol{X}}_{\boldsymbol{t}\boldsymbol{k}}'-{\boldsymbol{X}}_{\boldsymbol{t}\boldsymbol{b}}'\right| | (11) |
The ED time series in phase space is given by:
{\boldsymbol Z}=\left[{Z}_{1},\;{Z}_{2},\;\cdots ,\;{Z}_{n}\right] | (12) |
ED time series can reflect the stability change trend of measuring points. The instability of the filling body or rock mass is abrupt, and the distance between a phase point and its nearest phase point increases suddenly, thereby indicating that the measuring point is in an unstable state. ED has the following advantages: (i) the raw data for calculating the ED comes from various types of sensors, which can comprehensively reflect the stability of the system; (ii) it can effectively distinguish state changes before and after stability mutations; (iii) it is easily interpreted and understood by users.
In general, the ED time series of the filling body–surrounding rock combination is nonstationary. The ARIMA model is a combination of the differencing process, autoregressive (AR), and moving average (MA) methods [41]. The differencing process can effectively extract the certainty information in the nonstationary time series and convert it into a stationary time series. Using the AR and MA methods, the ARIMA model can deal with long time series with mutation characteristics.
The ARIMA model structure is defined as follows:
\left\{\begin{array}{l}\varPhi (B){\nabla }^{d}{Z}_{t}=\varTheta (B){\varepsilon }_{t}\\ E({\varepsilon }_{t})=0,\;\mathrm{Var}({\varepsilon }_{t})={\sigma }_{\varepsilon }^{2},\;E({\varepsilon }_{t}{\varepsilon }_{s})=0,\;s\ne t\\ E({Z}_{s}{\varepsilon }_{t})=0,\;\forall s < t\end{array}\right. | (13) |
where B is the delay operator; {Z}_{t} and {Z}_{s} are the ED value at time t and time s , respectively; {\varepsilon }_{t} and {\varepsilon }_{s} are the prediction error at time t and time s , respectively; {\nabla }^{d}={(1-B)}^{d} ; \varPhi (B)=1-{\phi }_{1}B-\cdots -{\phi }_{p}{B}^{p} , which is an autoregressive coefficient polynomial; \varTheta (B)=1-{\theta }_{1}B-\cdots -{\theta }_{q}{B}^{q} , which is an MA coefficient polynomial; \mathrm{V}\mathrm{a}\mathrm{r}({\varepsilon }_{t}) is the variance of the prediction error; {\sigma }_{\varepsilon } is the standard deviation of the prediction error.
The ARIMA model consists of three hyperparameters: p, d, and q. Here, d is the order in which the nonstationary time series needs to be differenced; p is the number of lags in the autoregressive method for processing the time series, indicating the relationship between the current value and the value at the past p time points; q is the number of lags in the prediction error of the MA method, indicating the relationship between the current value and the prediction error of the past q time points.
In this paper, we use the Augmented Dickey–Fuller test [42] to check the stationarity of the ED time series. If the ED time series is not stationary, it must be converted to a differential stationary time series. To avoid over-differentiation, we choose the minimum order that satisfies the conversion of ED time series to differential stationary time series as the model parameter d.
The values of p and q were determined based on the Akaike information criterion (AIC) [43] and the Bayesian information criterion (BIC) [44]. The evaluation indicators defined by these two criteria are respectively given by:
\mathrm{A}\mathrm{I}\mathrm{C}=2k-2\mathrm{l}\mathrm{n}(L) | (14) |
\mathrm{B}\mathrm{I}\mathrm{C}=\mathrm{l}\mathrm{n}(n)\cdot k-2\mathrm{l}\mathrm{n}(L) | (15) |
where k is the number of estimated parameters in the model; L is the maximum log-likelihood of model fitting; n is the number of data observed. The AIC and BIC values comprehensively consider the influence of model goodness of fit (i.e., log-likelihood) and model complexity (i.e., number of estimated parameters) on model performance. In this study, we select the minimum parameters p and q corresponding to the optimal AIC or BIC to avoid over-fitting of the model.
Root-mean-square error (RMSE) and relative root-mean-square error (rRMSE) are used to evaluate the prediction results of the model and can be calculated as follows:
\mathrm{R}\mathrm{M}\mathrm{S}\mathrm{E}=\sqrt{\frac{1}{N}\sum\nolimits_{i=1}^{N}{({Z}_{i}-{\hat{Z}}_{i})}^{2}} | (16) |
\mathrm{r}\mathrm{R}\mathrm{M}\mathrm{S}\mathrm{E}=\frac{{\mathrm{R}\mathrm{M}\mathrm{S}\mathrm{E}}_{\mathrm{P}\mathrm{r}\mathrm{e}\mathrm{d}\mathrm{i}\mathrm{c}\mathrm{t}\mathrm{i}\mathrm{o}\mathrm{n}}}{{\mathrm{R}\mathrm{M}\mathrm{S}\mathrm{E}}_{\mathrm{N}\mathrm{o}\mathrm{n}\mathrm{P}\mathrm{r}\mathrm{e}\mathrm{d}\mathrm{i}\mathrm{c}\mathrm{t}\mathrm{i}\mathrm{o}\mathrm{n}}}=\sqrt{\frac{\sum _{i=1}^{N}{({Z}_{i}-{\hat{Z}}_{i})}^{2}}{\sum _{i=1}^{N}{({\hat{Z}}_{i-{N}_{\mathrm{o}\mathrm{u}\mathrm{t}\mathrm{p}\mathrm{u}\mathrm{t}}}-{\hat{Z}}_{i})}^{2}}} | (17) |
where N is the number of measurement points; {Z}_{i} and {\hat{Z}}_{i} are the predicted and actual values in the same time point, respectively; {\mathrm{R}\mathrm{M}\mathrm{S}\mathrm{E}}_{\mathrm{P}\mathrm{r}\mathrm{e}\mathrm{d}\mathrm{i}\mathrm{c}\mathrm{t}\mathrm{i}\mathrm{o}\mathrm{n}} and {\mathrm{R}\mathrm{M}\mathrm{S}\mathrm{E}}_{\mathrm{N}\mathrm{o}\mathrm{n}\mathrm{P}\mathrm{r}\mathrm{e}\mathrm{d}\mathrm{i}\mathrm{c}\mathrm{t}\mathrm{i}\mathrm{o}\mathrm{n}} are the \mathrm{R}\mathrm{M}\mathrm{S}\mathrm{E} values calculated with the prediction and nonprediction methods, respectively; {N}_{\mathrm{o}\mathrm{u}\mathrm{t}\mathrm{p}\mathrm{u}\mathrm{t}} is the number of time points predicted by the model.
In nonprediction methods, the actual values {\hat{Z}}_{i} and {\hat{Z}}_{i-{N}_{\mathrm{o}\mathrm{u}\mathrm{t}\mathrm{p}\mathrm{u}\mathrm{t}}} are used to calculate the RMSE. Meanwhile, rRMSE highlights the performance of the selected prediction method in comparison to the nonprediction method. For example, when rRMSE = 0.6, we can infer that the prediction method can reduce the error by 40% compared with the error due to systematic delays. The smaller the rRMSE value, the greater the degree of the prediction method’s improvement relative to the nonprediction method.
We selected the data collected by the monitoring system of Longshou Mine in the past three years as the original dataset. The time series of each measuring point included the data from MDM, BSGs, and inclinometers.
The mutual information of data from different types of sensors may not reach the global (or local) minimum at the same time lag. Therefore, we selected the time lag corresponding to the minimum value of the average mutual information of all sensors in the measuring point as the parameter \tau . Fig. 5 illustrates the procedure employed to determine the time lag and embedding dimension for measuring point 1. The local minimum of the average mutual information is observed when the time lag is set to 9. When the embedding dimension is set to 6, the percentage of false nearest neighbor points is found to be below 5%. Table 3 presents a comprehensive overview of the time lag and embedding dimension associated with each measuring point used in the reconstruction of the phase space.
Measuring point ID | Time lag, τ | Embedded dimensions, d | Measuring point ID | Time lag, τ | Embedded dimensions, d | |
1 | 9 | 6 | 7 | 23 | 11 | |
2 | 1 | 4 | 8 | 14 | 2 | |
3 | 9 | 6 | 9 | 17 | 14 | |
4 | 11 | 11 | 10 | 25 | 11 | |
5 | 7 | 10 | 11 | 18 | 7 | |
6 | 19 | 2 | 12 | 2 | 5 |
Fig. 6 shows the ED time series evolution curve, the data measured by the sensors in situ, and the roadway repair amount of each measuring point. Based on the field investigation, several measuring points, such as Site 4, Site 5, Site 9, Site 10, and Site 11, experienced serious deformation at different times. The lengths of the roadway returns at these measurement points were 176, 178, 35, 28, and 43 m, respectively. The repairing events of the roadway are represented by pentagram symbols.
Given that the monitoring data came from several different types of sensors, they exhibited different trends. Therefore, changes in a single sensor time series cannot represent changes in the stability of the measurement point. At the same time, the time series of the sensors are heterogeneous, making it difficult to analyze the stability changes of a measurement point by directly observing the measured data.
Compared with measured data, ED time series can summarize data fluctuations from various types of sensors and highlight mutation events in the states of measurement points. At measurement points 6, 7, 8, and 12, even if the amount of variation in the measured data is small, mutation events in the stability state can still be recognized by observing the ED time series. The new method demonstrates a high sensitivity to changes in the stability state of the filling body–surrounding rock combination. We can also observe ED time series mutations at measurement points where no repairing event has occurred.
Multiple stability mutations were captured by evaluating distance time series at measurement points 5, 6, 11, and 12. The time period of the mutation in the ED time series corresponds to the process of stress changes and displacement occurrences in the filling body–surrounding rock combination. When the combination is in stress balance once again, the ED shifts to a stable time series until the next stability mutation event occurs.
The mutation of the ED time series indicates the occurrence of deformation and failure of the filling body–surrounding rock combination. Before each repairing event occurs, the ED time series is accompanied by a jump, and the start of these sudden change periods is generally 3–6 months earlier than the repairing time.
Due to the use of time lag in the process of phase space reconstruction, even short jumps in the data collected by the instruments can cause mutations in the ED time series at several consecutive time points. As a result, the changes in the stability of the filling body–surrounding rock combination are more easily captured. Objectively, the reliability of the EDs is mainly determined by the following aspects: (i) effective monitoring equipment and reasonable monitoring arrangement, which determine whether the raw data can reflect the stability changes in the monitored area; (ii) high-quality phase space reconstruction, which ensures that the intrinsic relevance of all raw data can be utilized to the maximum; (iii) continuous measurements over a long period of time, which helps to weed out short-term noise fluctuations.
To evaluate the performance of the ARIMA model in predicting the ED time series, the authors used ARIMA, LSTM, and Transformer models to analyze the same ED time series and compared the predicted value with the actual value.
The LSTM model is a time recurrent neural network with long-term memory [45]. Transformer is a deep learning model with self-attention and can assign different weights in accordance with the importance of each part of the input data [46]. Through hyperparameter tuning, the parameter settings of the LSTM and Transformer models are shown in Table 4.
Model | Hyperparameter | ||||||
LSTM | Input_size | Hidden_size | Num_layers | Train_epoch | Loss | ||
8 | 1 | 3 | 20 | Mean squared error | |||
Transformer | Input_size | d_model | n_heads | e_layers | d_layers | Train_epoch | Loss |
8 | 512 | 8 | 2 | 1 | 20 | Mean squared error |
Based on the ED time series of 12 measuring points, three models were used to predict the ED values of the next 300 time points. Referring to Eqs. (16) and (17), we calculated the RMSE and rRMSE of the prediction results of each model, respectively.
Fig. 7 shows the distribution of RMSE of the prediction results of the three models. As can be seen, the ARIMA and Transformer models exhibited a single-peak pattern with peak points of P1 = 0.26 and P2 = 0.37, respectively. The RMSE values for the different prediction steps of these two models are mainly concentrated around the peak points. The LSTM model is a bimodal form with the highest peak point at P3 = 0.50 and has a larger major distribution of RMSE values than the other two models. The RMSE of the ARIMA model prediction results is in a smaller range, followed by the Transformer model. The results reveal that the prediction accuracy of the ARIMA model is better than that of the other two models.
Fig. 8 shows the trend of RMSE of the prediction results of the three models with the number of prediction steps. As can be seen, the values of RMSE for the ARIMA and Transformer models remained stable over the range of prediction steps from 0 to 300. The RMSE value of the ARIMA model settled at 0.26 for the most part, with an extreme value of 0.89 at the jumping point. The Transformer model’s RMSE value was stable at 0.37 in the main but had some jump points. The maximum value of RMSE is 0.93. The LSTM model exhibited a robust upward trend within the prediction steps, and as the number of prediction steps increased, the RMSE value rose from 0.20 to 0.68, with a maximum value of 0.92 at the jumping point. Thus, the ARIMA model showed better performance in multistep prediction.
As shown in Fig. 9, the rRMSE values of the LSTM model show an increasing trend in the later part of the prediction period. As the number of prediction steps increased, the rRMSE value of the LSTM model increased from 1.03 to 5.25, with a local maximum of 9.43. Meanwhile, the rRMSE trends with the number of prediction steps are generally steady for the ARIMA and Transformer models. The ARIMA model rRMSE had a median of 0.83 and a great value of 5.30, while the Transformer model rRMSE had a median of 1.09 and a great value of 5.92. Thus, the ARIMA model had a smaller rRMSE value than the Transformer model.
When the rRMSE value was less than 1, the prediction model had better performance than the nonprediction method. Fig. 10 shows the rRMSE performance of the three models. The ratios of rRMSE less than 1 for Transformer, LSTM, and ARIMA models were 40%, 55%, and 70%, respectively. The results revealed that the ARIMA model had better prediction results than those of nonprediction methods in 70% of the cases.
In general, the deformation parameters, such as stress and displacement of the backfill–surrounding rock combination, remain stable until they are affected by tectonic stress or mining. The ED time series follows this rule; that is, the ED time series is stationary before the mutation occurs. Therefore, in the case of no mutation, the RMSE value of the nonprediction method is low, but it cannot effectively predict the occurrence of ED time series mutation. Upon comparing the RMSE and rRMSE values of the prediction results, we find that in the ARIMA model combined with the autoregressive and MA methods, the prediction results are closer to the historical average and have better performance in the prediction of ED time series.
This paper comprehensively evaluates the stability of the filling body–surrounding rock combination using heterogeneous information. The feasibility of the new method was verified in a downward-filling mine. The key findings of this work are enumerated below.
(1) The stability evaluation and prediction of backfill–surrounding rock combination is complex, dynamic, and uncertain. The data collected by the 3D monitoring system of filling body–surrounding rock combination constructed based on various monitoring equipment belong to heterogeneous information time series.
(2) The average mutual information method and the false nearest neighbor point method are used to determine the embedding dimension d and the delay time τ of the heterogeneous information phase space of the filling body–surrounding rock combination. The stability evolution law of the filling body–surrounding rock combination is fully demonstrated in the integrated time series.
(3) The distance between the phase point and its closest neighboring phase point in the phase space is proposed as the indicator ED to evaluate the changing amplitude of filling body–surrounding rock combination stability. In this case, the trend mutation of the ED time series indicates the deformation and damage of filling body–surrounding rock combination, which can identify the “key areas” affecting the stability of the mine.
(4) Comparing the accuracy of the ARIMA model with two deep learning models, namely LSTM and Transformer, in terms of predicting the ED time series, the ARIMA model has better performance than the others due to the combination of the autoregressive and MA methods.
This work was supported by the National Key R&D Program of China (No. 2022YFC2904103), the Key Program of the National Natural Science Foundation of China (No. 52034001), the 111 Project (No. B20041), and the China National Postdoctoral Program for Innovative Talents (No. BX20230041).
Shenghua Yin is an editorial board member for this journal and was not involved in the editorial review or the decision to publish this article. No potential conflict of interest was reported by the authors.
[1] |
S.H. Yin, Z.P. Yan, X. Chen, R.F. Yan, D.P. Chen, and J.W. Chen, Mechanical properties of cemented tailings and waste-rock backfill (CTWB) materials: Laboratory tests and deep learning modeling, Constr. Build. Mater., 369(2023), art. No. 130610. DOI: 10.1016/j.conbuildmat.2023.130610
|
[2] |
Z.L. Xue, H.K. Sun, D.Q. Gan, Z.P. Yan, and Z.Y. Liu, Wall slip behavior of cemented paste backfill slurry during pipeline based on noncontact experimental detection, Int. J. Miner. Metall. Mater., 30(2023), No. 8, p. 1515. DOI: 10.1007/s12613-023-2610-0
|
[3] |
L.H. Yang, J.C. Li, H.B. Liu, et al., Systematic review of mixing technology for recycling waste tailings as cemented paste backfill in mines in China, Int. J. Miner. Metall. Mater., 30(2023), No. 8, p. 1430. DOI: 10.1007/s12613-023-2609-6
|
[4] |
C. Hou, W.C. Zhu, B.X. Yan, K. Guan, and L.L. Niu, Analytical and experimental study of cemented backfill and pillar interactions, Int. J. Geomech., 19(2019), No. 8, art. No. 04019080. DOI: 10.1061/(ASCE)GM.1943-5622.0001441
|
[5] |
Q.H. Ma, G.S. Liu, X.C. Yang, and L.J. Guo, Physical model investigation on effects of drainage condition and cement addition on consolidation behavior of tailings slurry within backfilled stopes, Int. J. Miner. Metall. Mater., 30(2023), No. 8, p. 1490. DOI: 10.1007/s12613-023-2642-5
|
[6] |
Y.Y. Li, W.J. Guo, H.Q. Zhang, and Z.J. Wei, The application of combined GPS.RTK with electronic level on high-precision surface monitoring, Adv. Mater. Res., 718-720(2013), p. 1191. DOI: 10.4028/www.scientific.net/AMR.718-720.1191
|
[7] |
Y. Chen, J. Li, H.Z. Li, et al., Revealing land surface deformation over the Yineng backfilling mining area, China, by integrating distributed scatterer SAR interferometry and a mining subsidence model, IEEE J. Sel. Top. Appl. Earth Obs. Remote Sens., 16(2023), p. 3611. DOI: 10.1109/JSTARS.2023.3250419
|
[8] |
H.D. Fan, Q. Xu, Z.B. Hu, and S. Du, Using temporarily coherent point interferometric synthetic aperture radar for land subsidence monitoring in a mining region of Western China, J. Appl. Remote Sens, 11(2017), No. 2, art. No. 026003. DOI: 10.1117/1.JRS.11.026003
|
[9] |
C.D. Maio, G. Fornaro, D. Gioia, D. Reale, M. Schiattarella, and R. Vassallo, In situ and satellite long-term monitoring of the Latronico landslide, Italy: Displacement evolution, damage to buildings, and effectiveness of remedial works, Eng. Geol., 245(2018), p. 218. DOI: 10.1016/j.enggeo.2018.08.017
|
[10] |
W. Lei, S.J. Zhu, C. Jiang, et al., Research on 3D laser scanning monitoring method for mining subsidence based on the auxiliary for probability integral method, KSCE J. Civ. Eng., 25(2021), No. 11, p. 4403. DOI: 10.1007/s12205-021-0053-6
|
[11] |
Y.Y. Gu, D.W. Zhou, D.M. Zhang, K. Wu, and B.H. Zhou, Study on subsidence monitoring technology using terrestrial 3D laser scanning without a target in a mining area: An example of Wangjiata coal mine, China, Bull. Eng. Geol. Environ., 79(2020), No. 7, p. 3575. DOI: 10.1007/s10064-020-01767-1
|
[12] |
C. Liao, W.L. Luo, D.S. Cai, and M. Li, In situ measurement of rockfill dam settlement using fiber optic gyroscope monitoring system, Struct. Contr. Health Monit., 29(2022), No. 4, art. No. e2917.
|
[13] |
R.C.S.B. Allil, L.A.C. Lima, A.S. Allil, and M.M. Werneck, FBG-based inclinometer for landslide monitoring in tailings dams, IEEE Sens. J., 21(2021), No. 15, p. 16670. DOI: 10.1109/JSEN.2021.3081025
|
[14] |
C. Zhang, S.Y. Zhang, and J.Y. Cao, In-place fiber-optic inclinometer based on a vertical cantilever beam and dual FBGs, Opt. Laser Technol., 159(2023), art. No. 108933. DOI: 10.1016/j.optlastec.2022.108933
|
[15] |
Y.Q. Wang, S.B. Zhang, L.L. Chen, Y.L. Xie, and Z.F. Wang, Field monitoring on deformation of high rock slope during highway construction: A case study in Wenzhou, China, Int. J. Distrib. Sens. Netw., 15(2019), No. 12, art. No. 1550147719895953.
|
[16] |
V. Bennett, T. Abdoun, and M. Barendse, Evaluation of soft clay field consolidation using MEMS-based in-place inclinometer–accelerometer array, Geotech. Test. J., 38(2015), No. 3, p. 290. DOI: 10.1520/GTJ20140048
|
[17] |
D.W. Ha, J.M. Kim, Y. Kim, and H.S. Park, Development and application of a wireless MEMS-based borehole inclinometer for automated measurement of ground movement, Autom. Constr., 87(2018), p. 49. DOI: 10.1016/j.autcon.2017.12.011
|
[18] |
M. Khan, X.Q. He, J. Guo, and D.Z. Song, Accurate prediction of indicators for engineering failures in complex mining environments, Eng. Fail. Anal., 155(2024), art. No. 107736. DOI: 10.1016/j.engfailanal.2023.107736
|
[19] |
H.W. Jia, B.X. Yan, Z. Yang, and E. Yilmaz, Identification of goaf instability under blasting disturbance using microseismic monitoring technology, Geomech. Geophys. Geo Energy Geo Resour., 9(2023), No. 1, art. No. 142. DOI: 10.1007/s40948-023-00681-6
|
[20] |
X.G. Cheng, W. Qiao, and H. He, Study on deep learning methods for coal burst risk prediction based on mining-induced seismicity quantification, Geomech. Geophys. Geo Energy Geo Resour., 9(2023), No. 1, art. No. 145. DOI: 10.1007/s40948-023-00684-3
|
[21] |
T.W. Lan, X.T. Guo, Z.J. Zhang, and M.W. Liu, Prediction of microseismic events in rock burst mines based on MEA-BP neural network, Sci. Rep., 13(2023), No. 1, art. No. 9523. DOI: 10.1038/s41598-023-35500-1
|
[22] |
C.Y. Liu, G.H. Sun, X.X. Liu, et al., Construction of filling body instability failure warning model under single-side unloading condition, Rock Mech. Rock Eng., 55(2022), No. 7, p. 4257. DOI: 10.1007/s00603-022-02864-1
|
[23] |
Q. Yin, J.Y. Wu, C. Zhu, M.C. He, Q.X. Meng, and H.W. Jing, Shear mechanical responses of sandstone exposed to high temperature under constant normal stiffness boundary conditions, Geomech. Geophys. Geo Energy Geo Resour., 7(2021), No. 2, art. No. 35. DOI: 10.1007/s40948-021-00234-9
|
[24] |
Q.C. Ran, Y.P. Liang, Q.L. Zou, et al., Experimental investigation on mechanical characteristics of red sandstone under graded cyclic loading and its inspirations for stability of overlying strata, Geomech. Geophys. Geo Energy Geo Resour., 9(2023), No. 1, art. No. 11. DOI: 10.1007/s40948-023-00555-x
|
[25] |
L.M. Wang, X.Q. Zhang, S.H. Yin, X.L. Zhang, P.Z. Liu, and I.M.S.K. Ilankoon, Three-dimensional characterisation of pore networks and fluid flow in segregated heaps in the presence of crushed ore and agglomerates, Hydrometallurgy, 219(2023), art. No. 106082. DOI: 10.1016/j.hydromet.2023.106082
|
[26] |
H.J. Lu, Y.R. Wang, D.Q. Gan, J. Wu, and X.J. Wu, Numerical investigation of the mechanical behavior of the backfill–rock composite structure under triaxial compression, Int. J. Miner. Metall. Mater., 30(2023), No. 5, p. 802. DOI: 10.1007/s12613-022-2554-9
|
[27] |
W.Y. Cai, Z.C. Chang, D.S. Zhang, X.F. Wang, W.H. Cao, and Y.Z. Zhou, Roof filling control technology and application to mine roadway damage in small pit goaf, Int. J. Min. Sci. Technol., 29(2019), No. 3, p. 477. DOI: 10.1016/j.ijmst.2018.10.001
|
[28] |
S.H. Yin, Z.P. Yan, X. Chen, et al., Active roof-contact: The future development of cemented paste backfill, Constr. Build. Mater., 370(2023), art. No. 130657. DOI: 10.1016/j.conbuildmat.2023.130657
|
[29] |
M.L. Walske, H. McWilliam, J. Doherty, and A. Fourie, Influence of curing temperature and stress conditions on mechanical properties of cementing paste backfill, Can. Geotech. J., 53(2016), No. 1, p. 148. DOI: 10.1139/cgj-2014-0502
|
[30] |
M. Helinski, M. Fahey, and A. Fourie, Behavior of cemented paste backfill in two mine stopes: Measurements and modeling, J. Geotech. Geoenviron. Eng., 137(2011), No. 2, p. 171. DOI: 10.1061/(ASCE)GT.1943-5606.0000418
|
[31] |
M. Zhong, P. Yang, and Y.P. Hu, Study of instability mechanism and roof caving mode of cementing filling stope: The case study of a nonferrous metal mine in China, Adv. Civ. Eng., 2022(2022), art. No. 1658021.
|
[32] |
K. Ding, F.S. Ma, J. Guo, H.J. Zhao, R. Lu, and F. Liu, Investigation of the mechanism of roof caving in the Jinchuan nickel mine, China, Rock Mech. Rock Eng., 51(2018), No. 4, p. 1215. DOI: 10.1007/s00603-017-1374-0
|
[33] |
H.J. Zhao, F.S. Ma, Y.M. Zhang, and J. Guo, Monitoring and mechanisms of ground deformation and ground fissures induced by cut-and-fill mining in the Jinchuan Mine 2, China, Environ. Earth Sci., 68(2013), No. 7, p. 1903. DOI: 10.1007/s12665-012-1877-7
|
[34] |
G. Li, Y. Wan, J. Guo, F.S. Ma, H.J. Zhao, and Z.Q. Li, A case study on ground subsidence and backfill deformation induced by multi-stage filling mining in a steeply inclined ore body, Remote Sens., 14(2022), No. 18, art. No. 4555. DOI: 10.3390/rs14184555
|
[35] |
T.B. Zhao, Z.Y. Fu, and G. Li, In situ investigation into fracture and subsidence of overburden strata for solid backfill mining, Arab. J. Geosci., 11(2018), No. 14, art. No. 398. DOI: 10.1007/s12517-018-3769-y
|
[36] |
D.P. Chen, S.H. Yin, R.F. Yan, Y. Zhou, Y.F. Zhang, and L.M. Wang, State analysis of the inclinometer tube for monitoring relative slippage between backfill and surrounding rock mass, Int. J. Min. Reclam. Environ., 37(2023), No. 10, p. 856. DOI: 10.1080/17480930.2023.2245667
|
[37] |
A.M. Fraser and H.L. Swinney, Independent coordinates for strange attractors from mutual information, Phys. Rev. A, 33(1986), No. 2, p. 1134. DOI: 10.1103/PhysRevA.33.1134
|
[38] |
M.B. Kennel, R. Brown, and H.D. Abarbanel, Determining embedding dimension for phase-space reconstruction using a geometrical construction, Phys. Rev. A, 45(1992), No. 6, p. 3403. DOI: 10.1103/PhysRevA.45.3403
|
[39] |
H. Kantz and T. Schreiber, Nonlinear Time Series Analysis, Cambridge University Press, Cambridge, 2003.
|
[40] |
S. Wallot and D. Mønster, Calculation of average mutual information (AMI) and false-nearest neighbors (FNN) for the estimation of embedding parameters of multidimensional time series in Matlab, Front. Psychol., 9(2018), art. No. 1679. DOI: 10.3389/fpsyg.2018.01679
|
[41] |
G.E.P. Box and D.A. Pierce, Distribution of residual autocorrelations in autoregressive-integrated moving average time series models, J. Am. Stat. Assoc., 65(1970), No. 332, p. 1509. DOI: 10.1080/01621459.1970.10481180
|
[42] |
D.A. Dickey and W.A. Fuller, Distribution of the estimators for autoregressive time series with a unit root, J. Am. Stat. Assoc., 74(1979), No. 366a, p. 427. DOI: 10.1080/01621459.1979.10482531
|
[43] |
H. Akaike, A new look at the statistical model identification, IEEE Trans. Autom. Contr., 19(1974), No. 6, p. 716. DOI: 10.1109/TAC.1974.1100705
|
[44] |
G. Schwarz, Estimating the dimension of a model, Ann. Statist., 6(1978), No. 2, p. 461.
|
[45] |
S. Hochreiter and J. Schmidhuber, Long short-term memory, Neural Comput., 9(1997), No. 8, p. 1735. DOI: 10.1162/neco.1997.9.8.1735
|
[46] |
A. Vaswani, N. Shazeer, N. Parmar, et al., Attention is all you need, [in] NIPS'17 : Proceedings of the 31st International Conference on Neural Information Processing Systems, California, 2017, p. 6000.
|
[1] | Yao Huang, Wei-ning Liu, Ai-min Zhao, Jun-ke Han, Zhi-gang Wang, Hong-xiang Yin. Effect of Mo content on the thermal stability of Ti–Mo-bearing ferritic steel [J]. International Journal of Minerals, Metallurgy and Materials, 2021, 28(3): 412-421. DOI: 10.1007/s12613-020-2045-9 |
[2] | Homam Naffakh-Moosavy. Microstructural evolution and castability prediction in newly designed modern third-generation nickel-based superalloys [J]. International Journal of Minerals, Metallurgy and Materials, 2016, 23(5): 548-562. DOI: 10.1007/s12613-016-1266-4 |
[3] | Bo Song, Min Zhong, Xin Dong, Pei-fu Wang. Optimization of monitoring the stability of surrounding rock in a metal mine [J]. International Journal of Minerals, Metallurgy and Materials, 2009, 16(4): 359-367. DOI: 10.1016/S1674-4799(09)60065-0 |
[4] | Meifeng Cai, Shuhua Hao, Hongguang Ji. Regularity and prediction of ground pressure in Haigou Gold Mine [J]. International Journal of Minerals, Metallurgy and Materials, 2008, 15(5): 521-527. DOI: 10.1016/S1005-8850(08)60098-5 |
[5] | Chengxiang Yang, Zhouquan Luo, Guobin Hu, Xiaoming Liu. Application of a microseismic monitoring system in deep mining [J]. International Journal of Minerals, Metallurgy and Materials, 2007, 14(1): 6-8. DOI: 10.1016/S1005-8850(07)60002-4 |
[6] | Jun Wang, Chengchang Jia, Sheng Yin. Numerical prediction of the incremental melting and solidification process [J]. International Journal of Minerals, Metallurgy and Materials, 2003, 10(4): 50-54. |
[7] | K. Ohsasa, H. Shirosawa, T. Narita. Prediction of the solidification structure of casting and heterogeneous nucleation [J]. International Journal of Minerals, Metallurgy and Materials, 2003, 10(3): 43-46. |
[8] | Meifeng Cai, Jinan Wang, Shuanghong Wang. Prediction of Rock Burst with Deep Mining Excavation in Linglong Gold Mine [J]. International Journal of Minerals, Metallurgy and Materials, 2001, 8(4): 241-243. |
[9] | Qing Li, Deling Zheng, Jianlong Zhou. Stabilization of Chaotic Time Series by Proportional Pulse in the System Variable Based on Genetic Algorithm [J]. International Journal of Minerals, Metallurgy and Materials, 1999, 6(3): 228-229. |
[10] | Changxiang Xiang, Ningxin Zhang. Comparison of Some Methods on Prediction of Activity Interaction Coefficient [J]. International Journal of Minerals, Metallurgy and Materials, 1998, 5(1): 13-15. |
1. | Wenkai Ru, Diyuan Li, Hao Gong, et al. Numerical study on shear behavior of backfill-rock rough interface considering 3D morphology of surrounding rock in a mining stope. Computational Particle Mechanics, 2025. DOI:10.1007/s40571-025-00935-y |
2. | Qican Ran, Yunpei Liang, Zhili Yang, et al. Deterioration mechanisms of coal mechanical properties under uniaxial multi-level cyclic loading considering initial damage effects. International Journal of Rock Mechanics and Mining Sciences, 2025, 186: 106006. DOI:10.1016/j.ijrmms.2024.106006 |
Measuring points ID | Altitude / m | Location |
1 | 1500 | No. 22 exploration line |
2 | 1485 | No. 25 exploration line |
3 | 1470 | No. 28 exploration line |
4–6 | 1460 | No. 21, No. 25, and No. 33 exploration lines |
7–9 | 1280 | No. 14, No. 21, and No. 27 exploration lines |
10–12 | 1220 | No. 21, No. 25, and No. 29 exploration lines |
Instrument type | Number of installations | Description |
MDM | 1 | Two anchoring points |
BSG | 3 | Install in three mutually perpendicular directions |
Inclinometer | 1 | Inclinometer tube length 30–50 m |
Measuring point ID | Time lag, τ | Embedded dimensions, d | Measuring point ID | Time lag, τ | Embedded dimensions, d | |
1 | 9 | 6 | 7 | 23 | 11 | |
2 | 1 | 4 | 8 | 14 | 2 | |
3 | 9 | 6 | 9 | 17 | 14 | |
4 | 11 | 11 | 10 | 25 | 11 | |
5 | 7 | 10 | 11 | 18 | 7 | |
6 | 19 | 2 | 12 | 2 | 5 |
Model | Hyperparameter | ||||||
LSTM | Input_size | Hidden_size | Num_layers | Train_epoch | Loss | ||
8 | 1 | 3 | 20 | Mean squared error | |||
Transformer | Input_size | d_model | n_heads | e_layers | d_layers | Train_epoch | Loss |
8 | 512 | 8 | 2 | 1 | 20 | Mean squared error |